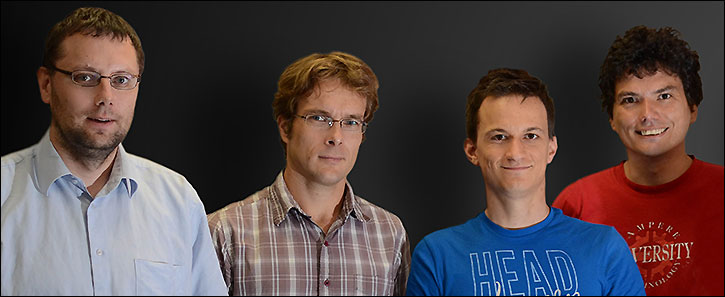
Vanderbilt team MarmotE cleared Phase 2 of the U.S. Defense Advanced Research Projects Agency’s Spectrum Collaboration Challenge held in December at the Johns Hopkins University Applied Physics Lab.
With no real estate left to expand the radio spectrum, DARPA’s challenge seeks machine-learning algorithms to sort out frequency priorities based on urgency – emergency and critical safety scenarios in real time – and to cooperate rather than compete for bandwidth.
By demonstrating that their AI could make more connections in a shared radio frequency spectrum environment without degrading other broadcasters in the process, MarmotE was one of six teams each awarded a $750,000 prize. The Vanderbilt team, which led 10 teams in Phase 1 competition in December 2017, came in second.
The money awarded to those six teams helps support the most promising designs, but the grand prize—$2 million—will go to the SC2 winner after a live competition at Mobile World Congress Americas in Los Angeles on Oct. 23, 2019.
MarmotE’s four team members, Peter Volgyesi, Miklos Maroti, Sandor Szilvasi and Peter Horvath, are current or former researchers and Ph.D. students working at the Vanderbilt Institute for Software Integrated Systems.
“Our team has a strong background in low-power multi-hop wireless network communication and time synchronization. Our approach is: ‘We question every assumption, have original ideas and can engineer a working and robust solution,’” said Volgyesi , a research scientist at the Vanderbilt Institute for Software Integrated Systems and a lecturer in the School of Engineering’s Department of Electrical Engineering and Computer Science.
Maroti, a former EECS research associate professor, is now an associate professor at the University of Szeged, Hungary. Szilvasi is a radio frequency and FPGA (field-programmable gate array) engineer in Atlanta. Horvath is an associate professor at Budapest University of Technology.
“Miklos is a mathematician, the main architect and the most active developer on our team,” said Volgyesi, the team’s lead. “Peter [Horvath] is a radio frequency and digital communication expert who offers theoretical support and Sandor helps in the performance evaluation of our design.”
“Our initial involvement with the Spectrum Challenge was motivated by National Science Foundation projects, NeTS: Experimental Platform for Low-Power Wireless Networking Research (#0964592), and Self-Sustaining CPS for Structural Monitoring (#1035627).
“In our NSF-NeTS project we were building a new low-power, software-defined radio platform called MarmotE. The ‘E’ stands for energy. The power savings is achieved with deep sleep periods of the node, hence the Marmot [large hibernating ground squirrel] part,” Volgyesi said.
The final challenge was a “payline” challenge. The goal was to prove not only that AIs could collaborate to manage spectrum, but also that it was more effective than the current method of chopping spectrum into defined bands. DARPA tallied points each AI earned across the more than 400 matches played by 15 teams.
Squeezing bits out of every hertz
Bandwidth is a finite resource and engineers are searching for ways to squeeze as many bits as possible out of every hertz. One way to avoid a spectrum crunch is to teach machines to be better at managing bandwidth than humans.
Instead of regulators chopping spectrum into defined, rigidly allocated bands, the hope is that devices can learn to autonomously manage their own spectrum use. That means training devices to listen for gaps in transmissions on viable frequencies, broadcast which frequencies they intend to use ahead of time and even negotiate with nearby devices that also need to send and receive signals.
The challenge comprised a series of matches representing real world scenarios such as managing transmissions from squads of soldiers deployed in an urban environment, or from Wi-Fi routers trying to deliver service despite a nearby radio jammer. Each AI scored points based on the number of connections it was able to deliver using the available spectrum—spectrum it was sharing with two, three or four other AIs at a time.
In one scenario, each AI represented an emergency response team deployed to combat a wildfire. The AIs had to work together to ensure each team’s radios and air tankers could communicate clearly on the same bands. The scenario was designed to test whether spectrum collaboration is viable in emergency, ad-hoc situations when manually re-allocating spectrum can take too long.
All tests were facilitated by the Colosseum, a 22-server emulator of real-world communications scenarios. In the system, 128 radios were connected directly to the emulator, which allowed SC2 participants to broadcast their signals directly into the machine. The Colosseum features 25.6 gigahertz of instantaneous bandwidth and can handle 420 terabits per second of digital radio frequency data.
In measuring data transmission speed, a terabit is one trillion binary digits, or 1,000,000,000,000 (that is, 1012) bits. A terabit is used for measuring the amount of data transferred in a second between two telecommunication points or within network devices.
“AI comes up with results that don’t make sense to humans,” said Paul Tilghman, DARPA’s project manager for SC2, in an article appearing in IEEE Spectrum. “But they work.”